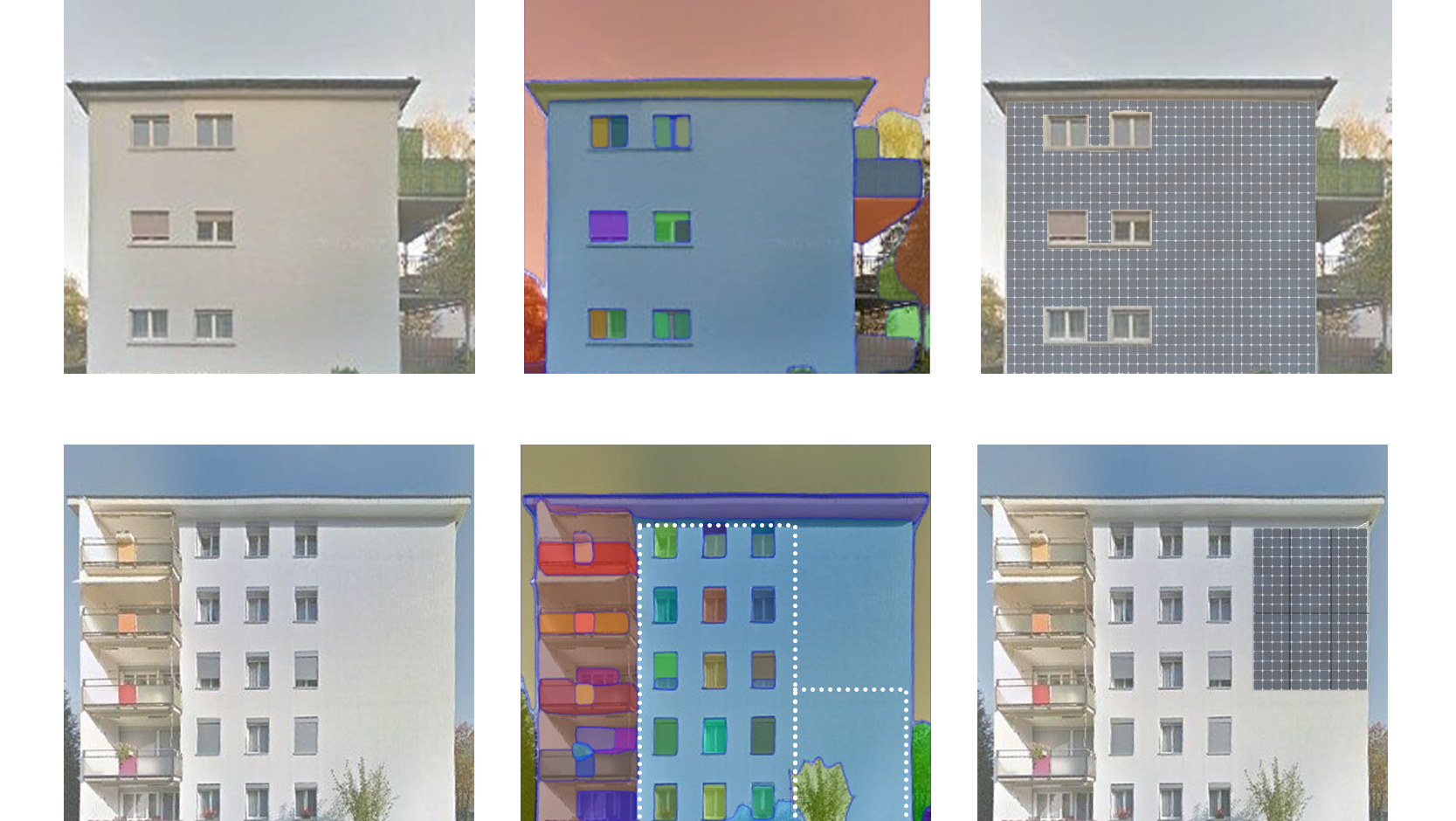
Segment4Solar v0.1
Segment for Solar Facades (S4S) is a deep learning–powered tool that analyzes building façade images to identify suitable areas for photovoltaic installation, integrating solar irradiance data to estimate energy yields with architectural detail.
2024
tool, research, deep learning
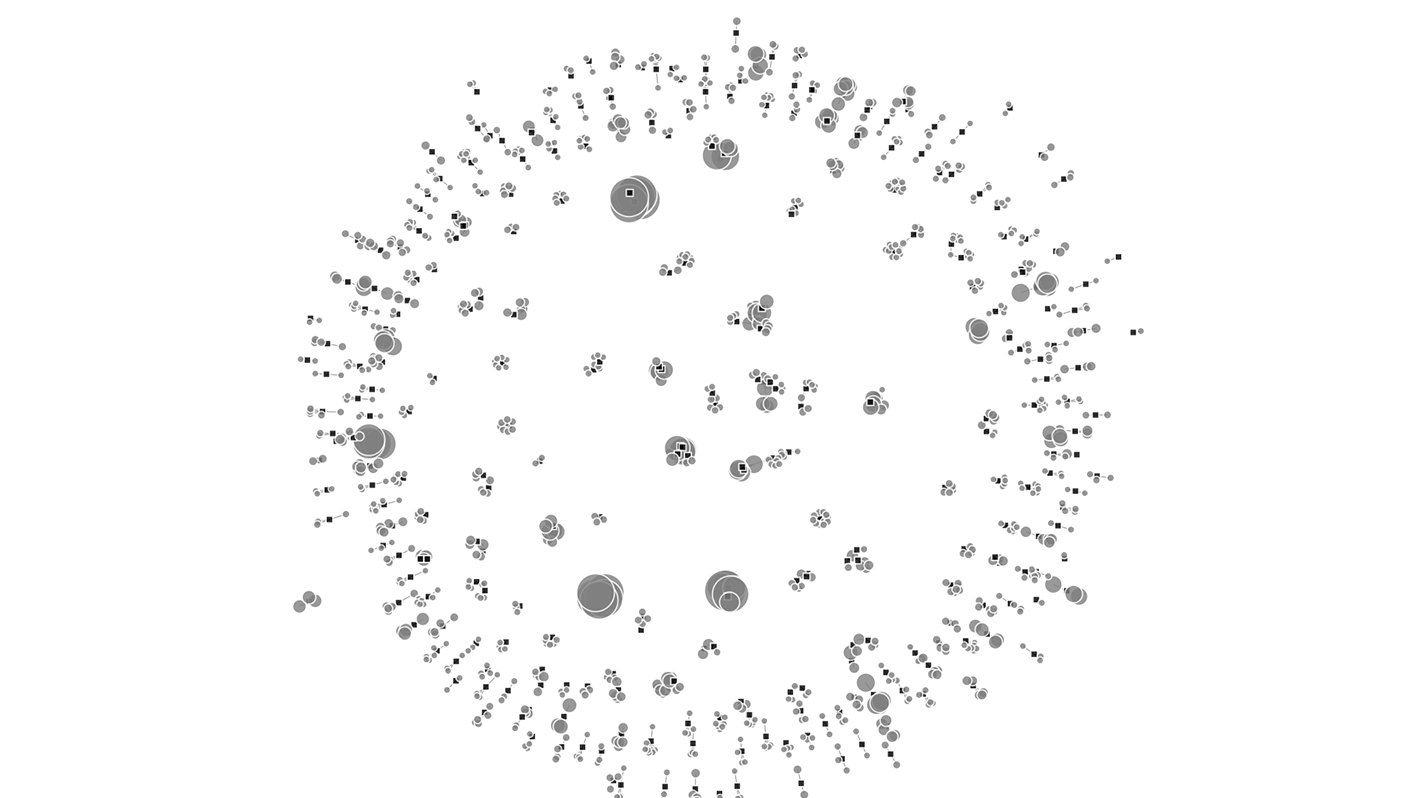
A review on AI applications for facades
This review analyzes trends and applications of AI methods—including Machine Learning, Deep Learning, and Computer Vision—in facade research, highlighting current uses, limitations, and future potential in architectural, environmental, and structural contexts.
2024
research, review, topic modelling
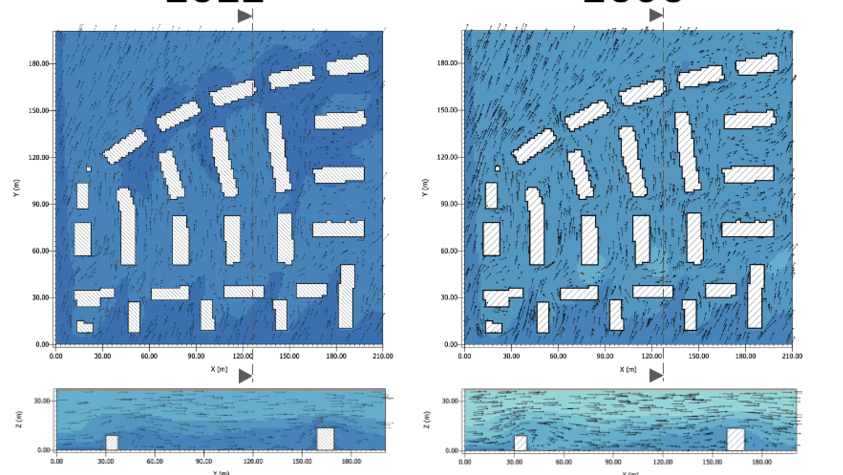
BIPV's impact on outdoor comfort in four block types
This study investigates how building-integrated photovoltaic (BIPV) façades affect outdoor thermal comfort across four urban block typologies in Zurich, revealing that urban form and climate change have a far greater impact than BIPV installation.
2024
research, simulation, urban design
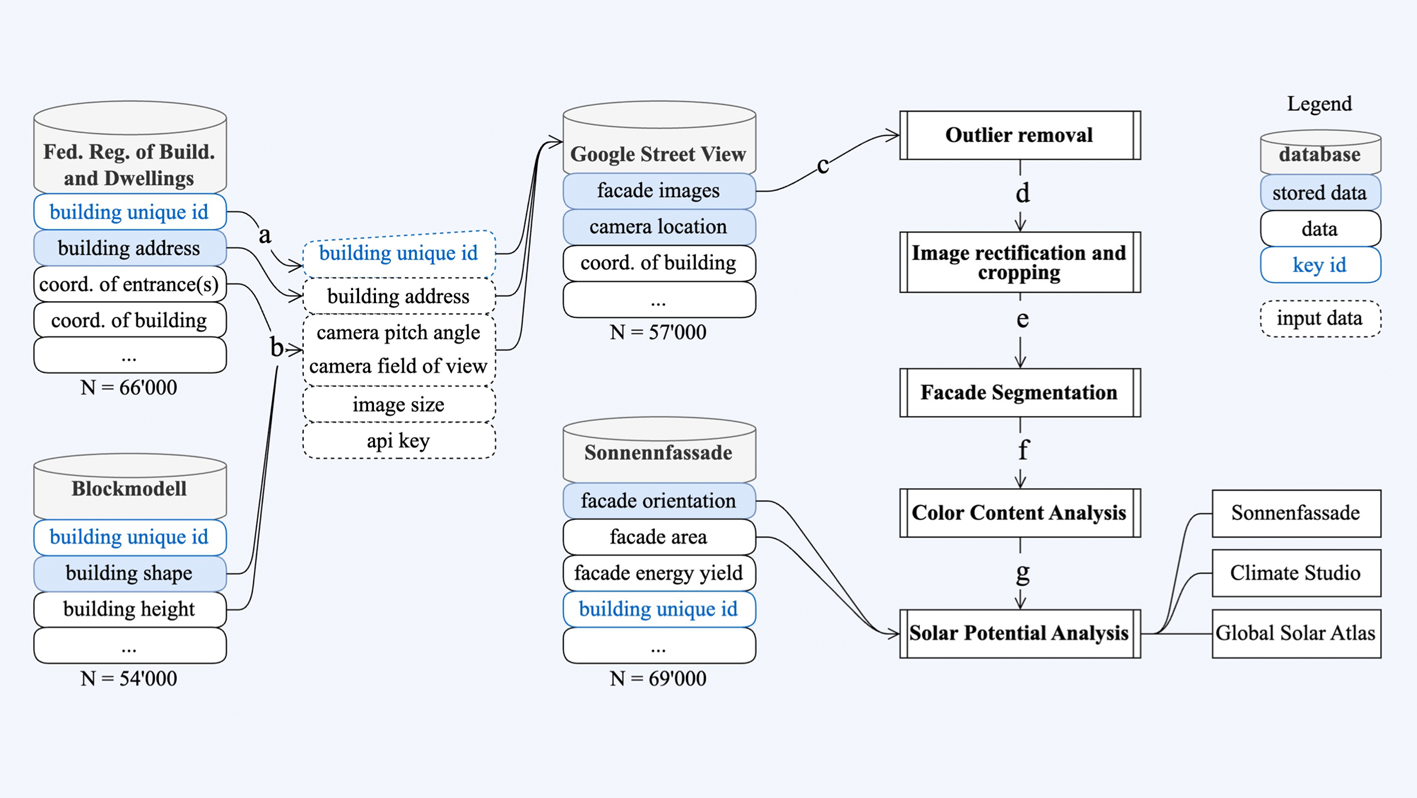
Facade feature extraction from open data sources
This work presents a method that combines open data sources and deep learning to extract detailed architectural façade features for more accurate estimation of BIPV energy potential.
2024
research, data scraping
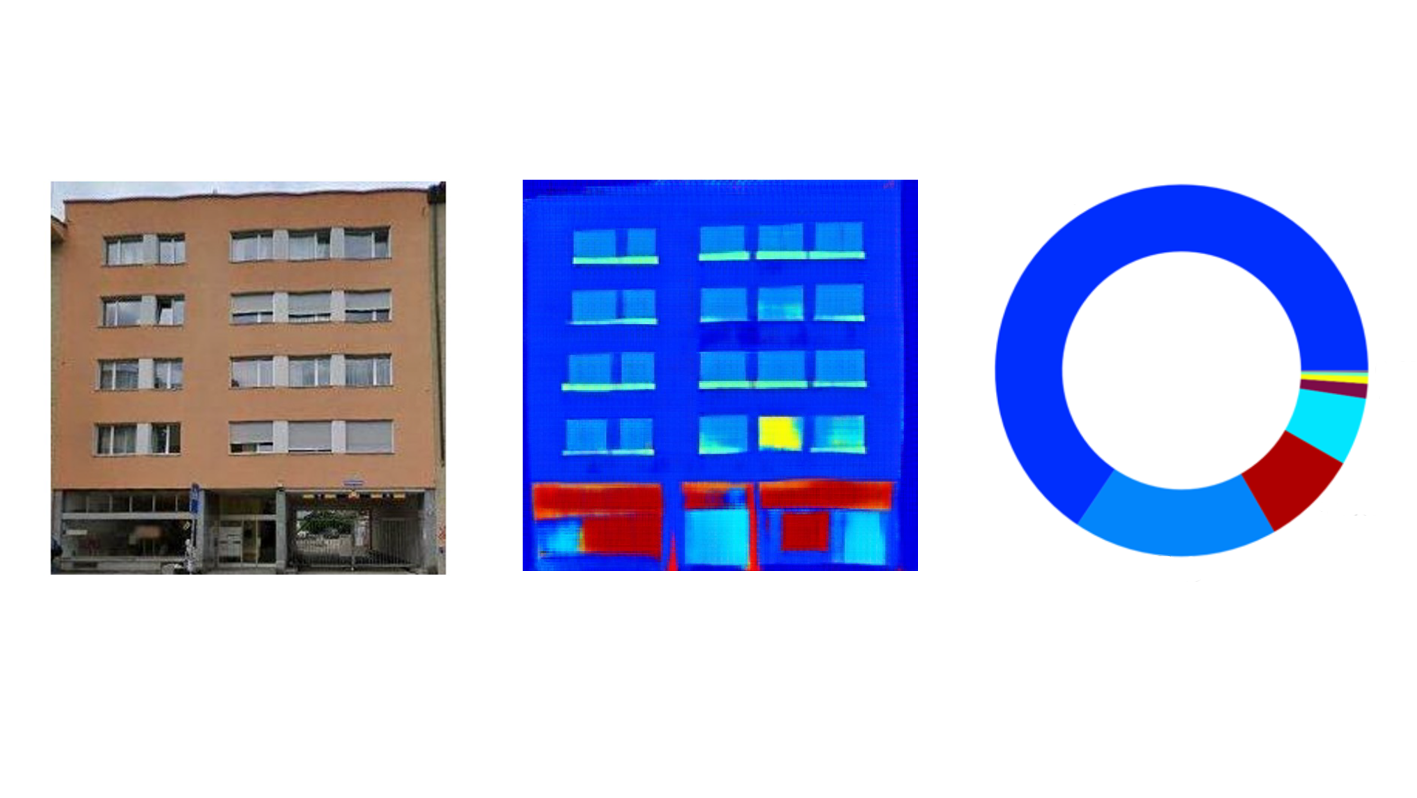
Deep learning‑based BIPV facade surface use estimation
This study introduces a deep learning–based method using GANs and street-level imagery to accurately estimate BIPV façade surface utilization, showing significant discrepancies with existing tools and emphasizing its potential to inform better solar energy planning and policy.
2023
research, deep learning
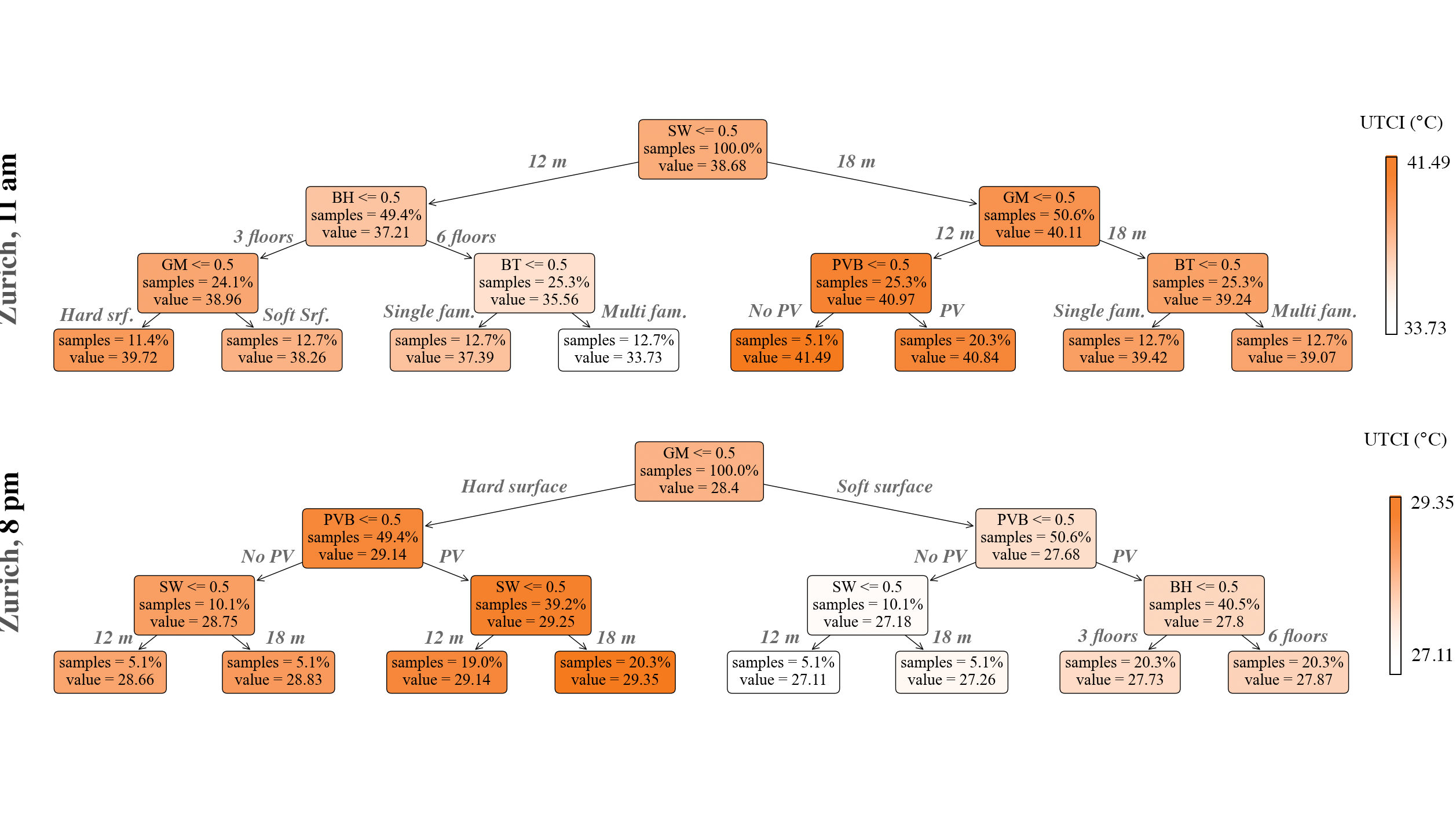
Parametric analysis of BIPV facades on outdoor comfort
This study uses a parametric simulation approach to assess how varying levels of BIPV façade deployment influence pedestrian-level outdoor thermal comfort across different urban settings, highlighting that factors like ground material and street width have a greater impact than BIPV itself.
2023
research, machine learning, simulation
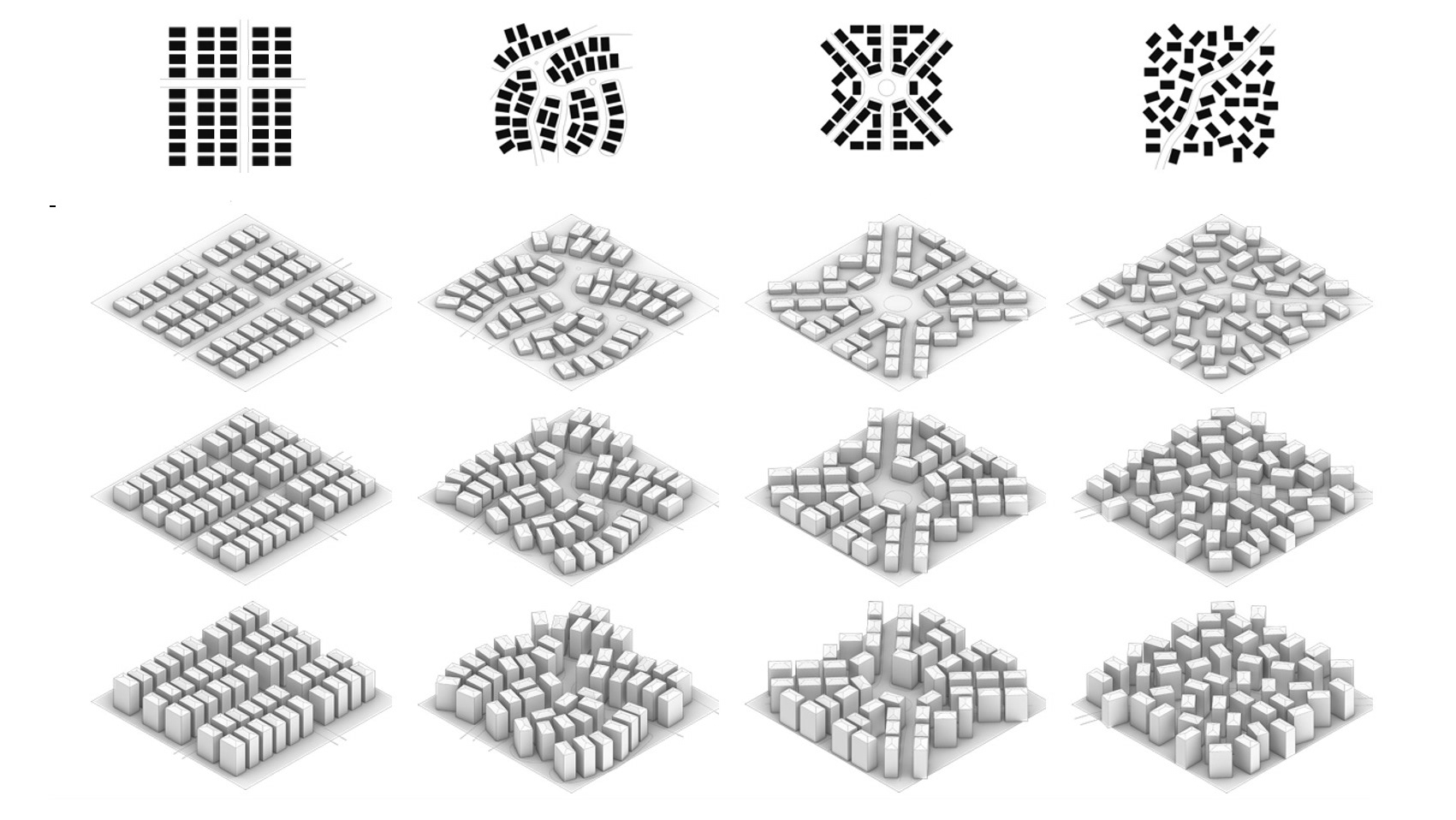
Urban Street Network and BIPV Generation Capacity
This study explores how different urban street network patterns influence the BIPV generation capacity of buildings, showing that irregular urban layouts enhance façade PV potential while roof PV potential remains largely unaffected by morphology.
2022
research, simulation, urban design
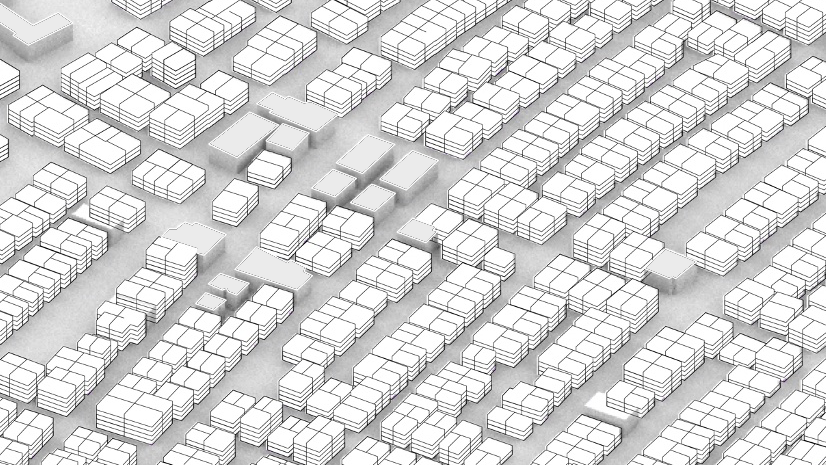
Compiling Open Datasets to Improve UBEM
This study enhances urban building energy models (UBEM) by integrating open data on occupancy and residential layouts, demonstrating that data-rich models significantly improve the accuracy of energy use and thermal comfort..
2022
research, data science, machine learning
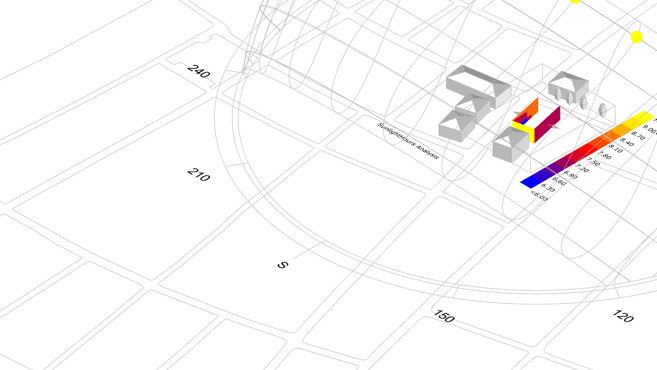
Solar Performance of Buildings in Urban Contexts
This thesis proposes a machine learning–based, data-driven method to predict BIPV generation potential on façades and roofs in diverse urban contexts, demonstrating high accuracy and highlighting the influence of urban morphology on solar performance.
2022
thesis, research, machine learning, simulation
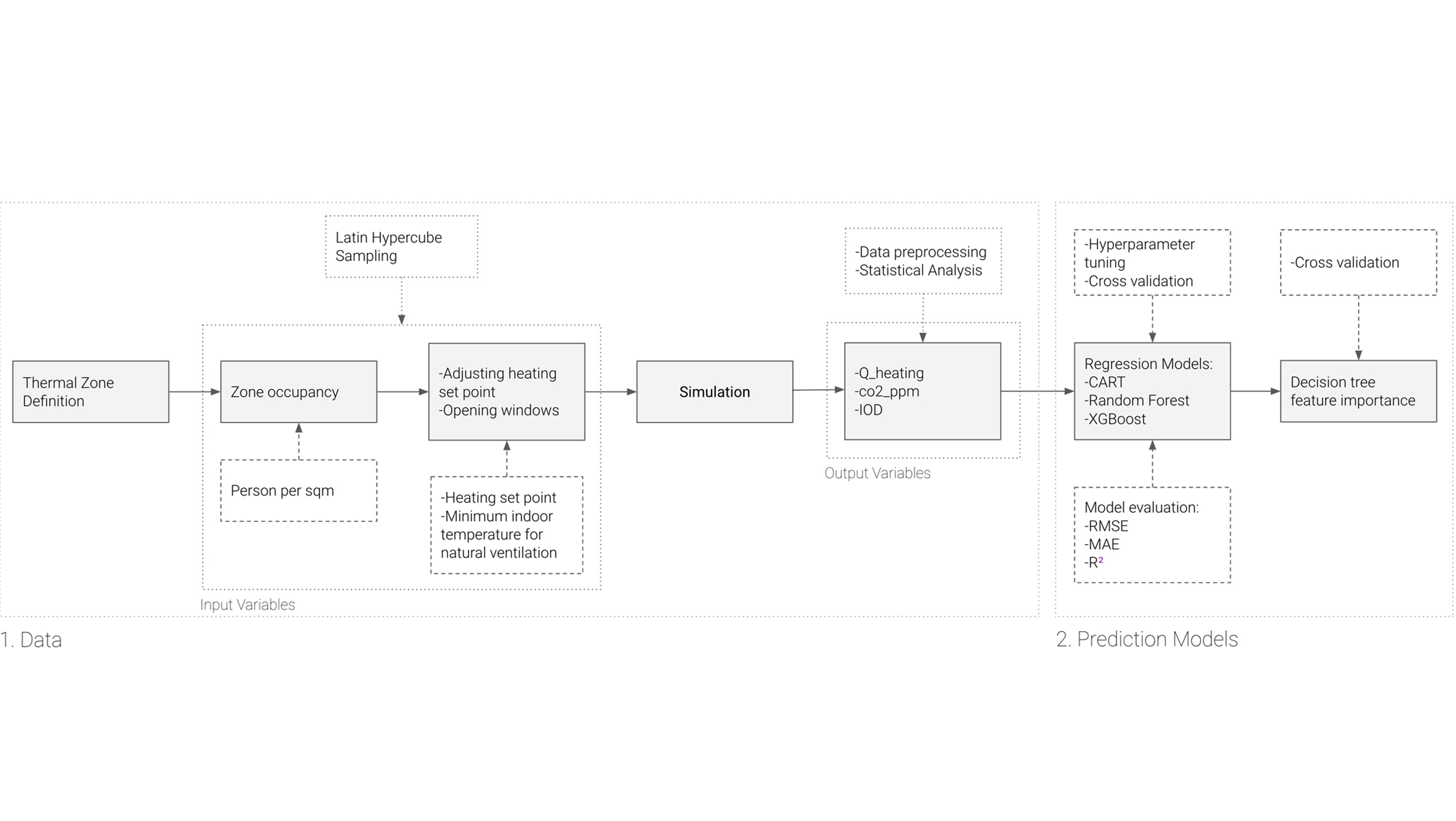
Data-driven Analysis of Impacts of Occupant Preferences
This study uses data-driven decision tree models to analyze how varying occupant thermal preferences impact comfort and energy performance in naturally ventilated classrooms, revealing key tradeoffs between occupant well-being and resource consumption in educational buildings.
2021
research, machine learning, simulation
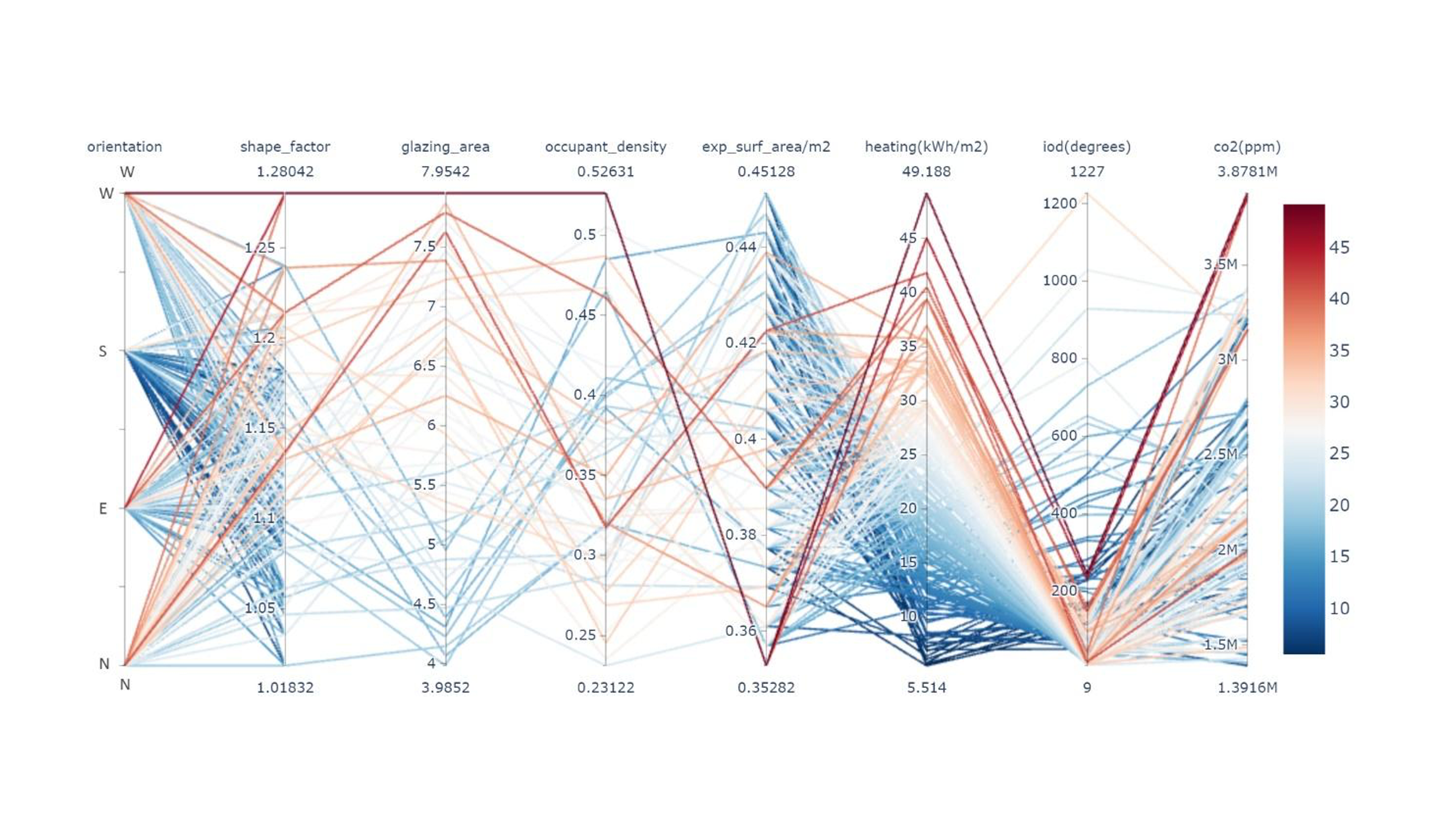
ANN to Predict Performance of Classroom Spaces
This study develops artificial neural network models to accurately predict heating demand, indoor overheating, and CO₂ levels in naturally ventilated classrooms, offering a fast, data-driven alternative to traditional simulations for evaluating classroom design and retrofit scenarios.
2021
research, machine learning, architectural design