A data-driven approach for predicting solar energy potential of buildings in urban fabric
Ayca Duran [Supervisors: Prof. Ipek Gursel Dino (architecture), Cagla Meral Akgul (civil eng.), Sinan Kalkan (computer science)]
Middle East Technical University, M.Arch. Thesis
Abstract Energy-efficient buildings that use clean and sustainable energy sources are urgently needed to reduce the environmental impact of buildings and mitigate climate change in cities. Buildings have great potential in harvesting solar energy by their solar exposure capacity. Developments in PV technologies also encourage the integration of PV systems into architectural applications. However, urban contexts can limit solar energy generation capacity of buildings by shading building envelopes and reducing available space for building-integrated PV (BIPV) deployment. Urban scale analyses are needed to understand the impact of urban form on solar energy generation potential. Nevertheless, urban scale analyses require tedious modeling and expertise, which may not be available during building design development or post-occupancy. This thesis presents a data-driven approach for predicting BIPV generation per façade and roof surface area utilizing machine learning (ML) techniques. As a first step, a simulation-based data generation method is applied to four neighborhoods in Ankara, representing different street network patterns. Three ML models are utilized and trained with simulation outputs. Two groups of predictors, simple and complex, are formed to allow predictions in various contexts. Overall, the best prediction model for facades has an R2 value of 0.98 and nRMSE of 10.83%. For roofs, R2 and nRMSE could reach up to 0.94 and 3.13%, respectively. Complex predictors offer better prediction performances, whereas basic predictors enable the prediction of BIPV potential independent of a 3D model. Varieties observed in prediction performances for different neighborhoods also imply the need for urban morphology inclusive approaches in predicting BIPV potential.
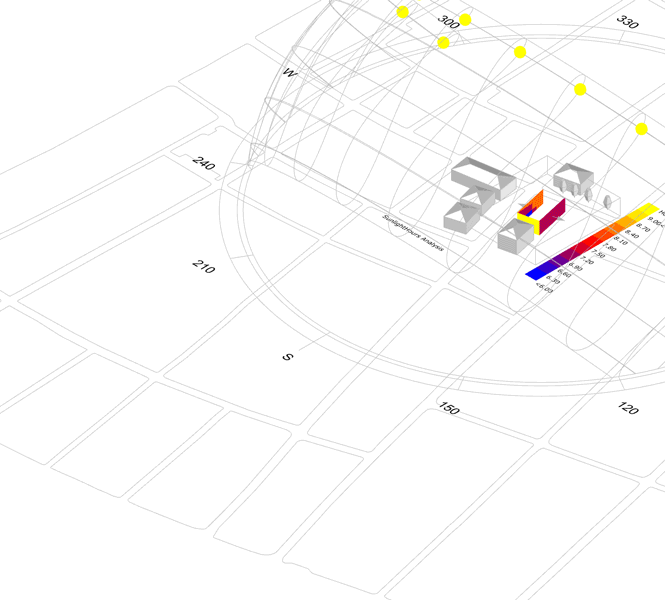
Citation
@mastersthesis{duran2022data,
title={A data-driven approach for predicting solar energy potential of buildings in urban fabric},
author={Duran, Ay{\c{c}}a},
year={2022},
school={Middle East Technical University}
}
title={A data-driven approach for predicting solar energy potential of buildings in urban fabric},
author={Duran, Ay{\c{c}}a},
year={2022},
school={Middle East Technical University}
}